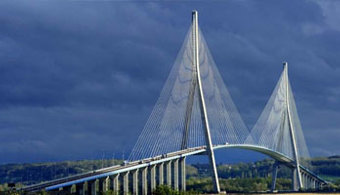
An in depth look at JF SME Testing's structural health monitoring solution for the Queensferry Crossing
Overview
Scotland’s Queensferry Crossing is one of Europe’s largest and most prestigious recent infrastructure projects. It is also one that defines a new global standard in embracing the latest big data, cloud computing, machine learning and internet of things technologies. The new bridge’s impressive Smart Asset Management System (SAMS) developed by JF SME Testing will deliver both a comprehensive real-time visibility of existing performance, and predictability of through-life needs that will help allow future maintenance to be more proactive, cost-efficient, and better targeted.
Spanning 2.7km and carrying the M90 motorway across the Firth of Forth, the Queensferry Crossing is the world’s longest three-tower, cable-stayed bridge. A significant impetus for the new bridge’s construction was the discovery in 2005/6 on the older Forth Road Bridge (FRB) of signs of deterioration, mainly as a result of the growth and increase in weight of traffic together with the influence of the weather and climate. Following inspection, severe corrosion was discovered, and the main suspension cables of the FRB were estimated to be working with a loss of strength of 8 to 10 per cent . Despite the measures taken to maintain the FRB and extend its useful lifetime, it was clear that it was not a viable long-term trunk route crossing. A new bridge – and a new approach to structural asset management – was clearly required.
Construction and specification
Opened in 2017, the Queensferry Crossing supersedes the FRB in carrying the M90 motorway and providing significantly improved capacity, while the older bridge is retained as a public transport, cycling and pedestrian corridor. Construction of the Queensferry Crossing was commenced in late 2011 by Forth Bridge Crossing Constructors (FCBC), a joint venture comprising Hochtief of Germany, American Bridge of Pittsburgh, United States, Dragados of Spain, and Morrison Construction of Scotland. JF SME Testing was contracted by this consortium in 2013 to provide a comprehensive structural health monitoring system.
Much more than a conventional structural health monitoring system, however, the solution provided by JF SME Testing for the new Queensferry Crossing would provide a fundamental leap forward in terms of technology. The highly innovative smart asset management system (SAMS) solution developed by JF SME Testing is a world first: much wider in scope and ambition than any conventional real-time structural health monitoring system, it draws on some of the latest technologies in big data, the internet of things sensor fusion, advanced statistical analysis, and cluster computing in order to better enable the structure’s current and future managers to predict maintenance and to target resources as efficiently as possible. In short, the SAMS solution developed by JF SME Testing for the Queensferry Crossing moves the science of structural health monitoring forward from one of immediate operational decision support, problem identification and reactive maintenance, to one that facilitates a more proactive and resource-efficient approach to the through-life management and operation of a structure.
Real-time sensors and processing
Monitoring a structure as complex as the Queensferry Crossing requires a large number of sensors, delivering a vast amount of data in real time. At the core of the SAMS solution developed by JF SME Testing for its client, FCBC, is the BridgeWatch® software, which acts as the kernel of the real-time structural health monitoring system. This is configured with advanced data acquisition and processing capabilities and amalgamates data from almost 1900 permanent sensor channels.
The sensor network is linked to 45 data acquisition unit (DAU) ‘outstations’ distributed along the bridge. These are connected via a fibre optic network to a server at one abutment, and to the bridge control office. Each of the DAUs receives input data from static and dynamic strain gauges, displacement sensors of various types, environmental sensors including anemometers, barometers, rainfall gauges, air temperature and relative humidity sensors, structure temperature sensors for the steel, concrete, asphalt and stay cables, and accelerometers to monitor the motion of the structure.
At hardware and software levels, the solution incorporates three specialist monitoring systems which have been integrated with BridgeWatch® in order to combine the information obtained into a seamless user interface environment. These are GPS receivers for position monitoring of towers and deck, a dynamic weigh-in-motion system for traffic load monitoring and alerts, and a corrosion monitoring system for reinforced concrete at splash zones of towers and selected piers.
Monitoring, analysis, and ‘Digital Twins’
Combined and analysed within BridgeWatch®, these data provide the bridge operators with an instantaneous vision of the performance of the structure (both as measured via the sensors or via indirect derivation) as it responds to operational traffic loadings and prevailing meteorological conditions. Immediate information is available, therefore, to support operators in decision-making on traffic load management and maintenance operations.
The ‘open architecture’ nature of the system is demonstrated by its ability to integrate virtual sensors and structural models in an approach that JF SME Testing terms the BridgeWatch® ‘Digital Twin’. The Digital Twin comprises one or more parts of the structure which are represented by detailed 3D finite element models. Using sensor data for displacements and other structural loads and responses, and automatically correlating and validating the predicted strains and displacements with measured sensor data, the Digital Twin models can thus provide a highly detailed and real-time resolution of stress and strain distribution throughout the regions modelled. The Digital Twin concept thus enables a more informed and detailed representation than previously possible, enabling operators to home in on any structural detail and assess its response to real-time traffic loadings and significant weather events. By focusing on regions of suspect structural detailing – or where problems are already known to occur – the approach enables a far better understanding of performance and the likely prognosis for any issues found.
Importantly, the system enables this level of analysis and ‘virtual sensor’ placement to extend to regions of the structure where physical monitoring would difficult, impossible, prohibitively expensive or potentially hazardous for operatives engaged in the installation or maintenance of physical sensor systems. Using Digital Twin, operators can thus look inside the structure at any instant, observing stress concentrations and potential damage locations. In situations where incipient failures have been detected, the approach enables advanced non-linear analyses – for example, to predict crack propagation or to assess the likely effectiveness of remedial repair options.
The Digital Twin also benefits from the technology-agnostic approach adopted by JF SME Testing, meaning that input data may be obtained from any source or external provider. This extends to the incorporation of inspection records for key structural components, including photographs and videos. Records can be entered via a handheld device and automatically uploaded with a time-stamp and GPS location via BridgeWatch®. These then form a part of the real-time through-life data record of the structure, together with the sensor data and Digital Twin analysis results. While extremely useful in the context of a structural health monitoring application, the open architecture approach of the SAMS/BridgeWatch® system is even more powerful when exploiting the potential of big data technologies and machine learning artificial intelligence to predict and assess future performance.
Sheer volume of data
With a large number of sensor channels providing readings in real-time, the volume of data generated by the SAMS/BridgeWatch® system is vast. While many current state-of-the-art solutions are capable of real-time analysis, fully harnessing the power of such a detailed through-life digital record of a structure is a far more challenging problem. With its 1900 sensor channels, the derived data channels from the system’s analytical processes, plus with multiple additional sources – from visual inspection records to Digital Twin models – a structure such as the Queensferry Crossing SAMS solution can generate multiple terabytes of data per week. It is arguable that civil engineering lags behind other sectors in its ability to manage and utilise the benefits of big data, and in making full use of internet of things innovations which generate data at this level. Data may be recorded, but beyond initial real-time analysis, the process in most current applications tends to be one of merely logging and archiving.
To harness the power of big data technology in the Queensferry Crossing SAMS system, JF SME Testing utilised open, web-based technologies that are readily available and proven in sectors as disparate as online gaming, video-on-demand services, international banking and automated share market trading. Similarly, the analysis functions of the system harness the type of parallel processing approaches used in sectors such as automotive and aerospace for large, highly non-linear analysis operations.
Big data processing systems are typically assembled using range of sophisticated open software technologies in the form of a so-called ‘technology stack’. The technology stack includes the operating system and related support programs and all runtime environments necessary to support a given application, as well as database warehousing software and utilities for version control. For an application such as the SAMS/BridgeWatch® system, modules are required to enable and manage distributed and massively parallel computing, so that flexible resource can be utilised far beyond the scope of the more traditional single server-based solutions. To envisage the modular nature of the technology stack for a given application, it may be helpful to imagine it as being comprised of Lego bricks – each representing one of the constituent open technologies and collectively building towards the required overall analytical processing capabilities and data warehousing capacity and structure.
The approach enables the rapid development of analytical systems based on highly complex processing operations, as the raw technology development is effectively pooled across multiple applications in multiple industrial sectors. It also enables data architectures to be preserved and new functionality to be added as and when it becomes available throughout the life of the structure. The term ‘open’ is not, however, indicative of risk – the same rigorous security protocols used in applications such as banking are available to protect such big data innovations.
Machine learning and predicting future performance
Using these big data technologies, the SAMS/BridgeWatch® system enables far more advanced forms of structural health monitoring to be carried out. By harnessing flexible, web-accessible parallel computing resources, highly sophisticated analysis can be used. For example, the system can be configured to automatically execute sophisticated analysis operations based on a fixed schedule, or according to pre-set data triggers such as threshold exceedances. These can be either individual sensor inputs such as a given load, temperature, displacement or wind speed for a given direction, or a prescribed combination of such inputs or derived parameters such as bending moment. Alternatively, analyses may be triggered on-demand or from another analytical process.
Machine learning routines can be configured to predict future performance trends based on the complex data sets ranging from displacements to traffic loadings, temperatures and wind speeds. In a highly complex system such as a major suspension bridge, assessing subtle trends in the vast quantity of multi-sensor data generated, can be extremely challenging. The use of deep learning neural network methods can enable multiple layers of nonlinear information processing for unsupervised self-learning performance based on large amounts of sensor derived data. Advanced analysis routines can be used to automatically calculate trends in the expected future performance deterioration of structural details of concern.
Any divergence between the predicted performance and real-time measured data, or an increase in the predicted level of structural deterioration could be used in itself as a trigger for further analysis and investigation. One such analysis operation that might be used on this through-life data is that of stochastic subspace identification (SSI). For a welded structure, for example, there will be a known overall stiffness on completion. If a fracture occurs in part of the structure the stiffness will be changed, but it may not be possible to spot this in the complexity of recorded data, due to other factors such as prevailing temperatures, wind speeds and traffic loadings. SSI enables the numerical manipulation of systems where not all variables are known, in order to help identify and isolate the causes of deterioration based on the live loadings applied.
Early detection of root causes for even comparatively incipient problems should thus be identifiable, enabling early rectification or even preventative action to be taken in a planned way, thus avoiding the costs and disruption of more intensive interventions that might otherwise be required at a later stage of deterioration.
Construction monitoring – capturing key data for later use
In addition to the obvious benefit of designing-in the SAMS/BridgeWatch® system from the outset in a way that ensures access to all of the requisite monitoring sensors, communications and processing, this approach by JF SME Testing also allowed for a unique, one-off opportunity to record crucial structural performance data relating to the construction process. For example, monitoring was carried out to record the build-up of permanent load in each of the concrete towers during their construction and the process of lifting each of the deck sections into place. As a part of this process, the compression and bending of each tower was observed arising from the eccentricity of loading represented by each deck lifting operation. In effect, each stage of this one-time construction loading provides a unique datum against which future structural response can be correlated.
An object lesson in the need for structural health monitoring
Even while the SAMS/BridgeWatch® system was being developed and installed on the Queensferry Crossing, its forerunner in carrying the M90 motorway across the Firth of Forth provided a text book example of why structural health monitoring is desirable for such critical national infrastructure. In December 2015 the Forth Road Bridge was closed to traffic for a number of weeks as an emergency measure while major repairs were carried out, following the discovery of fractures resulting from a seized pin in one of the crucial truss end links. Such was the cost and disruption caused, that an inquiry was held by the infrastructure and capital investment committee of the Scottish Parliament. Questions were asked as to whether the presence of a structural health monitoring system would have enabled the problem to have been recognised at an earlier stage and managed at less cost and disruption . Had a system of the type installed on the Queensferry Crossing been available on the older bridge, it is indeed highly likely that the problem would have been foreseen and proactively managed without the disruption caused.
Ongoing through-life monitoring of Queensferry
The Queensferry Crossing has thus provided a case study on how the civil engineering industry can utilise some of the latest internet of things and big data technologies to more proactively manage structures throughout their operational life. When fully commissioned and handed over, the SAMS/BridgeWatch® system will provide the operators of this important transport link with a flexible and user-friendly software interface available to control room staff, and a sophisticated database of structural load and response data that will grow and develop throughout the many decades of the structure’s life. Most importantly, the technology stack allows for the advanced analysis and machine learning capabilities, that will enable the bridge operators to manage the structure in a proactive manner, so as to avoid the unexpected issues of deterioration that beset the older Forth Road Bridge. Being open technology, the system will be upwards compatible with even more powerful big data and complex analysis innovations that will surely arrive in the years ahead.
- Read article
Detection of bridge impact
Read articleBridgestrike installed two key bridges London underground
Read articleJF SME Testing delivers structural health monitoring project three Normandy bridges
Read articleJF SME Testing commissions structural health monitoring solution Queensferry crossing
Read articleQueensferry crossing meets internet things new bridge new rules
Read articleBridge load testing eco city, Kuala Lumpur
Read articleHidden bridge defects | Sub structure solutions
Read article